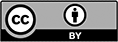 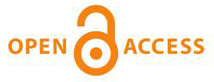
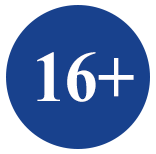
Юридический и почтовый адрес учредителя и издателя: САФУ им. М.В. Ломоносова, наб. Северной Двины, д. 17, г. Архангельск, Россия, 163002
Адрес редакции: «Вестник САФУ. Серия "Гуманитарные и социальные науки"», ул. Урицкого, 56, г. Архангельск
Тел: (818-2) 21-61-00, вн. 18-20
Сайт: https://vestnikgum.ru
e-mail: vestnik_gum@narfu.ru
|
Possible use of remote sensing for reforestation processes in Arctic zone of European Russia. P. 106–113
|
|
Section: Geosciences
Download
(pdf, 0.5MB )
UDC
630*1
DOI
10.3897/issn2541-8416.2018.18.3.106
Authors
NA Demina 1, AA Karpov 1,2, VV Voronin 1, EV Lopatin 1, AP Bogdanov 1,2
1 Northern Research Institute of Forestry (Arkhangelsk, Russian Federation)
2 Northern (Arctic) Federal University named after Lomonosov (Arkhangelsk, Russian Federation)
Corresponding author: Alexander A. Karpov ( xxstpatrickxx@gmail.com)
Abstract
This article considers the possibility of using remote sensing to monitor reforestation as exemplified in the
Severodvinsk and Onezhsk forestry districts of the Arkhangelsk region of Russia’s Arctic zone. Remote sensing
makes use of medium spatial resolution satellite images and high resolution unmanned aerial vehicle
(UAV) images. In the course of work on the project, a preliminary method was developed for reforesting
land previously subjected to cutting, fire, or windfall. Steps include detecting a reduction in forest cover and
collecting field data through the use of UAVs to create a training set, which is used to classify satellite images
according to the two classes of ‘restored’ or ‘not restored’. Various data processing tools are used to perform
these steps. The Tasseled Cap multi-channel satellite image transformation method is employed as a tool for
detecting a reduction in forest cover and analysing reforestation. The k-nearest neighbour algorithm is employed
to classify satellite images. This article provides a step-by-step algorithm for monitoring and an assessment
is provided of the situation in relation to forest regeneration in the Severodvinsk and Onezhsk forestry
districts. The work carried out has shown that it is possible to use UAV images to monitor forest recovery,
which is of significant importance for the conditions of the Arctic zone of European Russia.
Keywords
reforestation, forest monitoring, forest cutting, forest dynamic, boreal forest, Landsat, Sentinel, remote sensing, ERS, unmanned aerial vehicle, UAV
References
- Fadaei H, Sakai T, Yoshimura T, Kazuyuki M (2010) Estimation of tree density with high resolution imagery in the Zarbin forest of North Iran (Cupressus sempervirence var. Horizontalis). International Archives of the Photogrammetry, Remote Sensing and Spatial Information Sciences, ISPRS Congress, Kyoto, Japan, XXXVIII, Part 8, 679–684.
- Fiorella M, William J (1993) Ripple analysis of Conifer forest regeneration using landsat thematic mapper data. Photogranunetric Engineering & Remote Sensing 59(9): 1383–1388.
- Franks S, Masek JG, Turner MG (2013) Monitoring forest regrowth following large scale fire using satellite data – A case study of Yellowstone National Park, USA. European Journal of Remote Sensing 46: 561–569. https://doi.org/10.5721/Eu-JRS20134632
- Frazier RJ, Coops NC, Wulder MA (2015) Boreal shield forest disturbance and recovery trends using Landsat time series. Remote Sens. Environ. 170: 317–327.https://doi.org/10.1016/j.rse.2015.09.015
- Healey SP, Cohen WB, Zhiqiang Y, Krankina ON (2005) Comparison of tasseled cap-based Landsat data structures for use in forest disturbance detection. Remote Sensing of Environment 97: 301–310. https://doi.org/10.1016/j.rse.2005.05.009
- Huang C, Wylie B, Yang L, Homer C, Zylstra G (2001) Derivation of a Tasseled Cap transformation based on Landsat 7 at-satellite reflectance. Raytheon ITSS, USGS EROS Data Centre Sioux Falls, SD 57198, 10 pp.
- Kauth RJ, Thomas GS (1976) The Tasseled Cap – A Graphic Description of the Spectral-Temporal Development of Agricultural Crops as Seen by LANDSAT. Proceedings of the Symposium on Machine Processing of Remotely Sensed Data, Purdue University of West Lafayette, Indiana, 41–51.
- Krankina O, Cohen W, Yang Zh (2005) Method for Monitoring Forest Regrowth with Satellite Imagery. 31st International Symposium on Remote Sensing of Environment, Global Monitoring for Sustainability and Security, June 20–24, Saint-Petersburg. http://www.isprs.org/proceedings/2005/ISRSE/html/welcome.html
- Maltese A, Blanca B, Ciraolo G, Cox E, Loggia G, La Mela DS (2005) Veca A post-fire analysis of vegetation dynamics in semiforested areas using multi-temporal remote sensing imagery. In: Olsson H (Ed.) ForestSat 2005 in Borås May 31 – June 3. Proceedings of Rapport, 23–27.
- Sperlich M, Kattenborn T, Koch B, Kattenborn G (2014) Potential of Unmanned Aerial Vehicle Based Photogrammetric Point Clouds for Automatic Single Tree Detection. DGPF Proceedings; Gemeinsame Tagung 2014 der DGfK, der DGPF, der GfGI und des GiN, DGPF Tagungsband 23 / 2014.
- Vorobev ON, Kurbanov EA, Polevshchikova YuA, Lezhnin SA (2016) Assessment of the dynamics and disturbance of forest cover in the Middle Volga region using Landsat images. Contemporary Issues of Remote Sensing of the Earth from Space 13(4): 124–134.
- Wallace L, Lucieer A, Watson C, Turner D (2012) Development of a UAV-LiDAR System with Application to Forest Inventory. Remote Sensing 4: 1519–1543. https://doi.org/10.3390/rs4061519
- Yu Q, Gong P, Clinton N, Biging N, Kelly M, Schirokauer D (2006) Object-based detailed vegetation classification with airborne high spatial resolution remote sensing imagery. Photogrammetric Engineering & Remote Sensing 72: 799–811. https://doi.org/10.14358/PERS.72.7.799
|